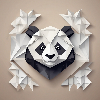
What is the best algorithm for predicting crypto currency?
I am interested in predicting the crypto currency market. I want to know which algorithm is the most effective for making accurate predictions in this domain.
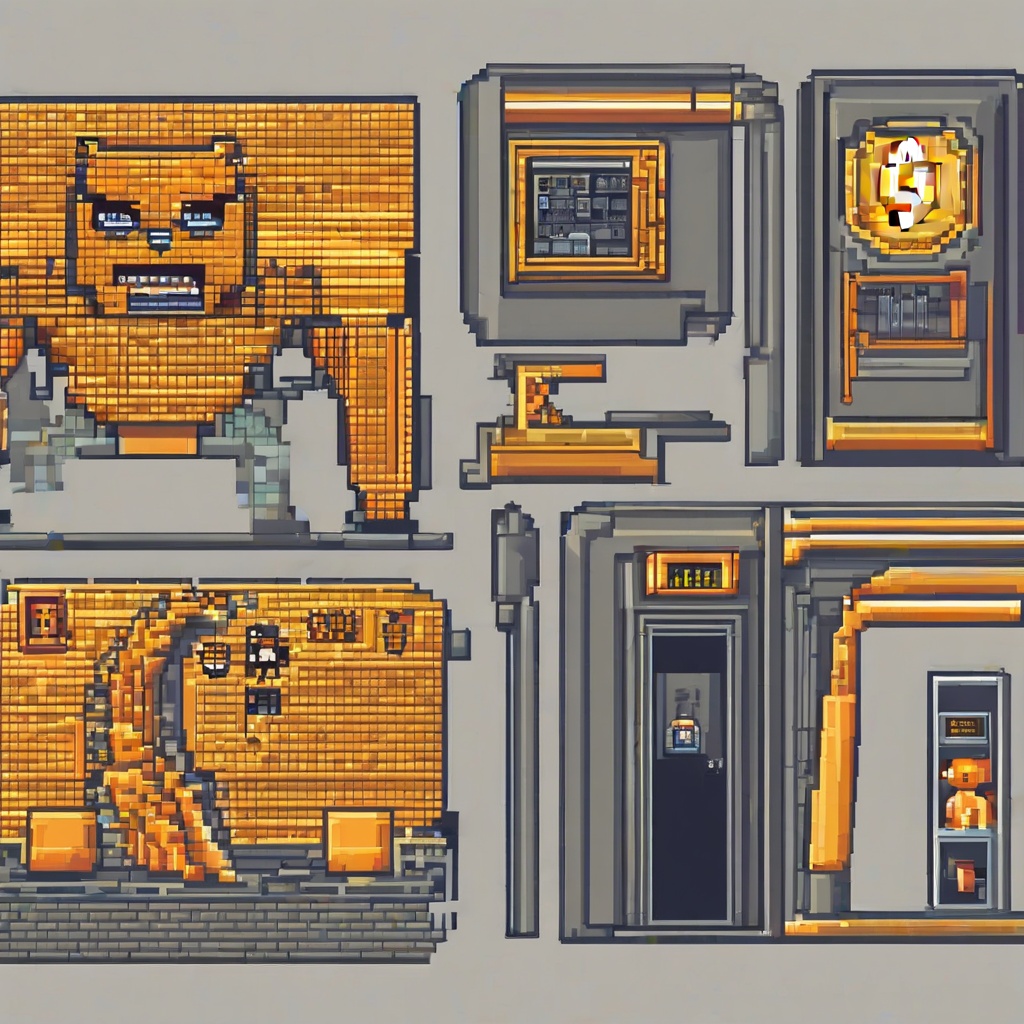
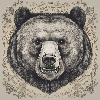
Is tarot predicting future?
I'm curious about tarot cards and their ability to predict the future. I've heard people say they can see what's coming based on these cards, but I'm not sure if it's really true. Can tarot actually predict the future?
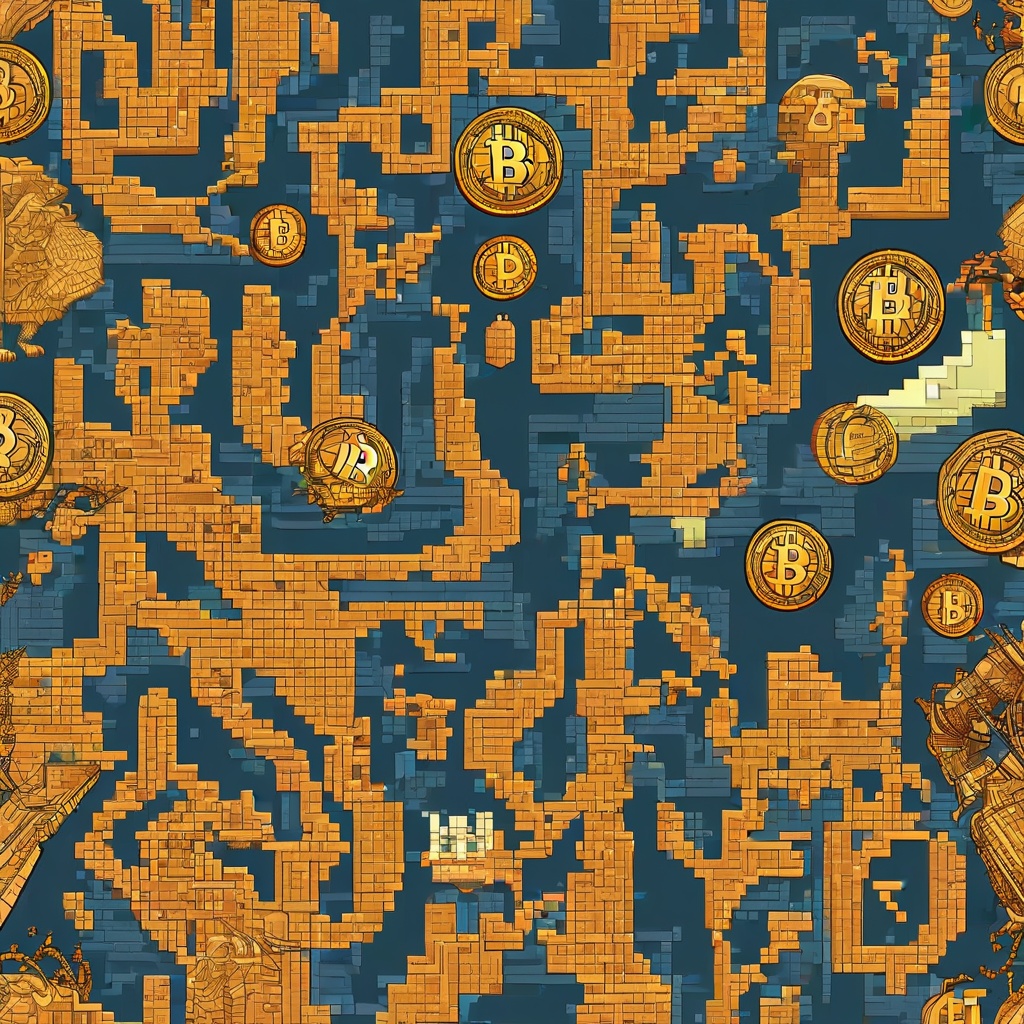
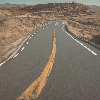
Which machine learning model is best for predicting crypto prices?
It's a fascinating question to ponder upon: which machine learning model stands out as the most effective in forecasting cryptocurrency prices? With the ever-fluctuating market and a myriad of variables at play, choosing the right model is crucial. Do deep learning algorithms, with their ability to extract intricate patterns from vast amounts of data, hold the key? Or could it be more traditional models like linear regression, which offer simplicity and interpretability? The search for the optimal solution involves weighing factors such as accuracy, computational efficiency, and the ability to adapt to changing market conditions. Ultimately, the answer may lie in a blend of models, tailored to suit the unique characteristics of the cryptocurrency market.
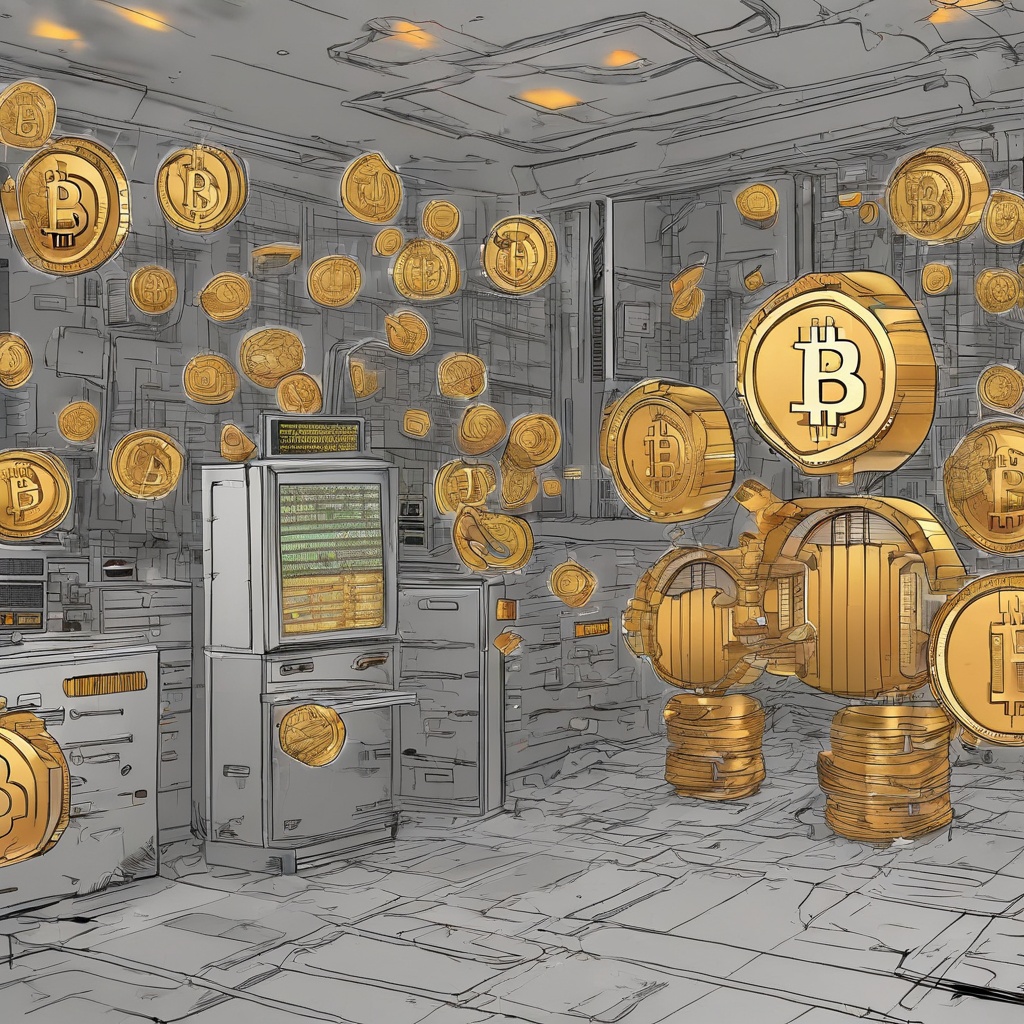
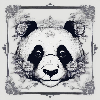
How accurate is S2F for predicting bitcoin price?
In the realm of cryptocurrency forecasting, the Stock-to-Flow (S2F) model has gained significant attention for its purported ability to predict Bitcoin's price movements. However, the question remains: How accurate is S2F in truly capturing the intricate dynamics of the Bitcoin market? Critics argue that the model oversimplifies the complex economic factors that influence Bitcoin's value, while proponents highlight its historical accuracy in forecasting key price points. This begs the question: Is S2F a reliable tool for predicting Bitcoin's future price, or does it merely offer a theoretical framework that often fails to account for real-world variables? The answer lies in a careful analysis of the model's assumptions, its historical track record, and its limitations in a rapidly evolving market.
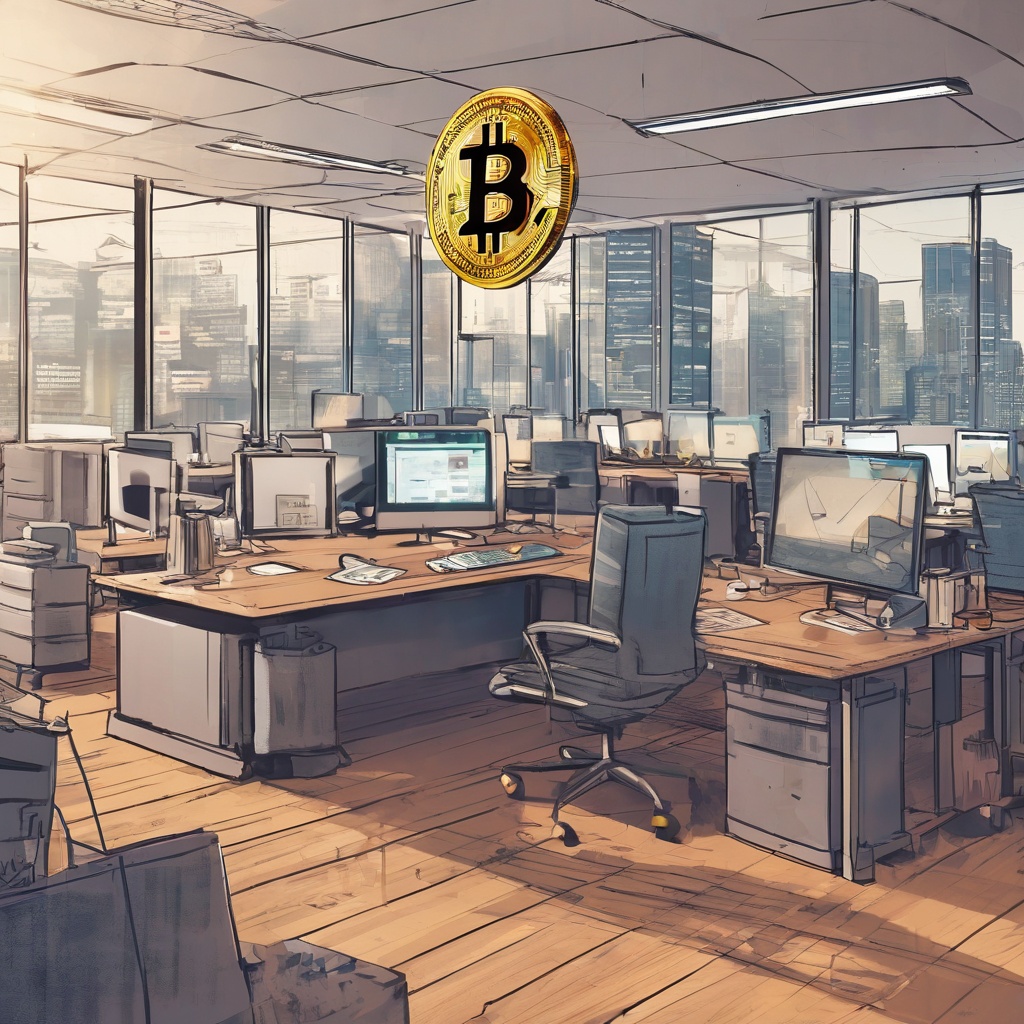