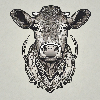
What is the difference between probit and logit?
Can you explain the key differences between probit and logit models in the context of econometrics and finance? I'm curious about how they are applied, the underlying assumptions they make, and any limitations they may have in comparison to each other. Also, when would you recommend using one over the other when analyzing financial data or cryptocurrency markets?
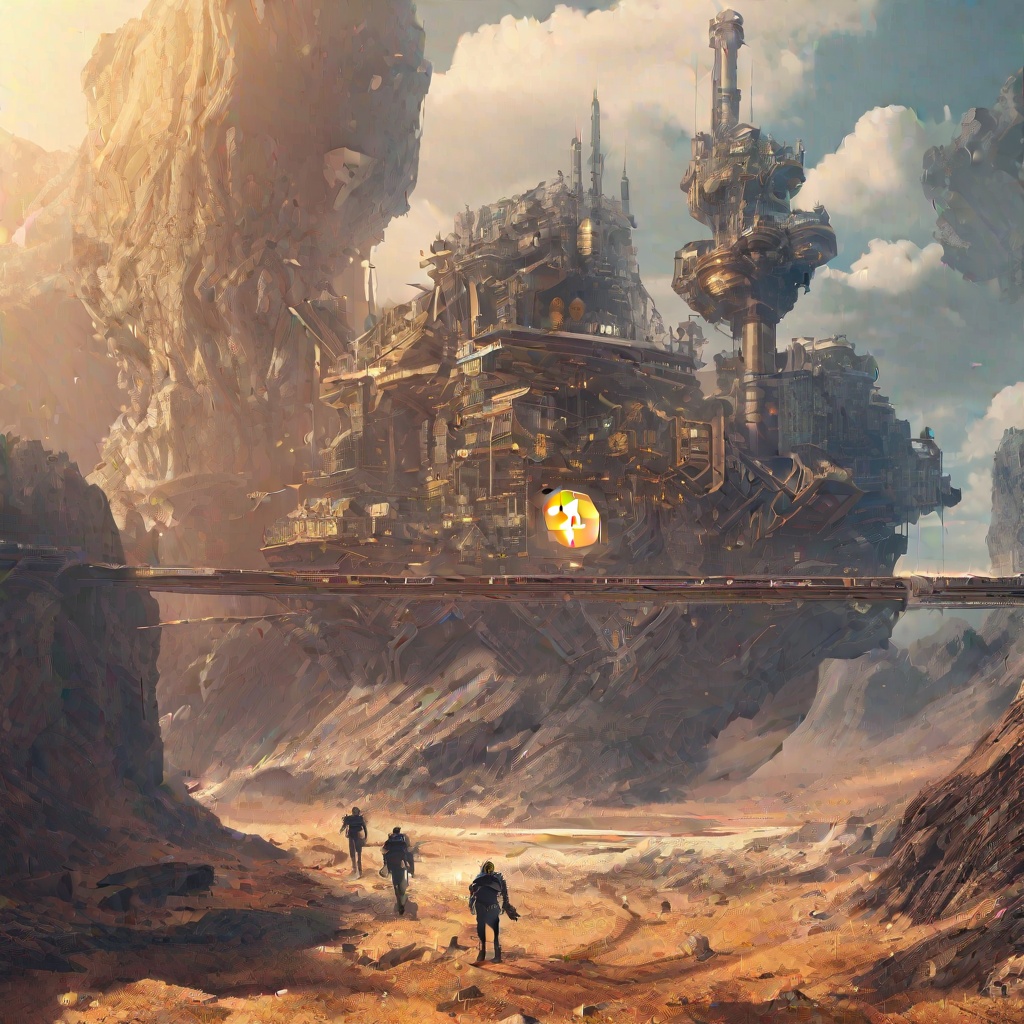
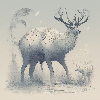
Why is logit called a regression?
I'm curious to understand why the term "logit" is referred to as a regression, despite its seemingly distinct mathematical and statistical underpinnings. Could you elaborate on the rationale behind this classification, highlighting the key similarities or connections that justify labeling logit as a regression model, especially in the context of statistical analysis and modeling?
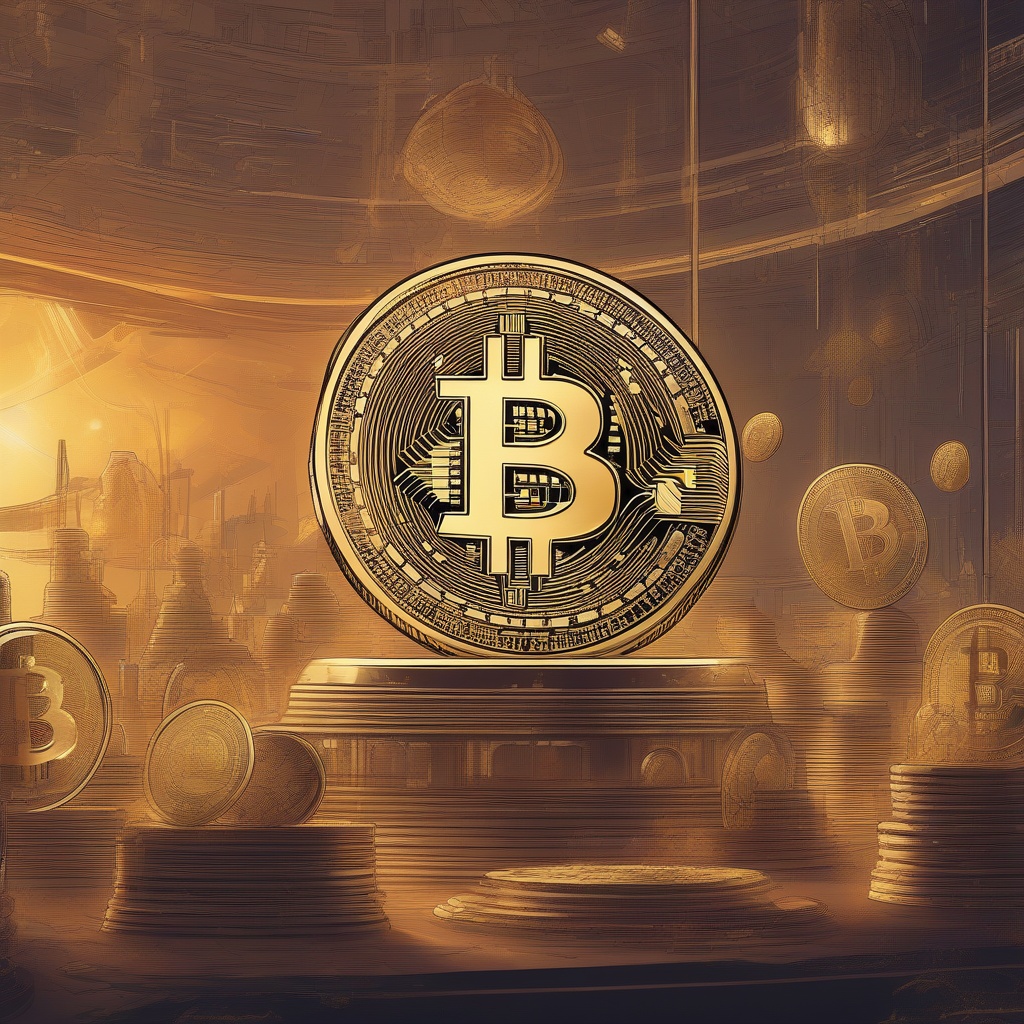
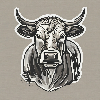
When to use probit vs. logit?
In the realm of statistical modeling, the choice between probit and logit models can often be a perplexing one. So, when should one opt for a probit model over a logit model, and vice versa? What factors should be taken into consideration to make an informed decision? Is there a specific type of data or research question that leans more towards one model than the other? As a researcher or analyst, how can one ensure that their choice aligns with the assumptions and characteristics of each model?
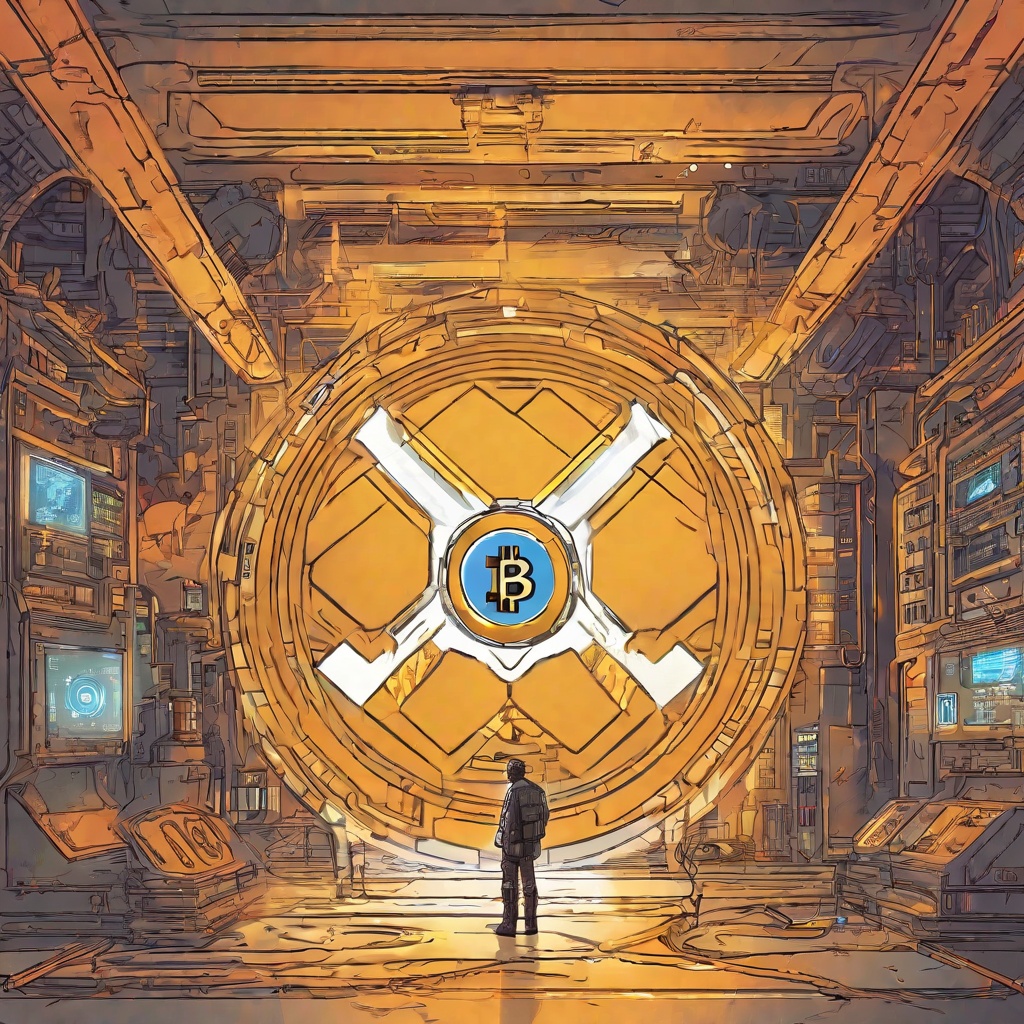
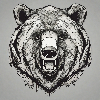
Is propensity score matching logit or probit?
Could you please clarify whether propensity score matching is typically done using a logit or probit model? I understand that both logit and probit models are used in regression analysis for binary outcomes, but I'm not sure which one is more commonly applied in the context of propensity score matching. Is there a specific reason why one might be preferred over the other in this scenario?
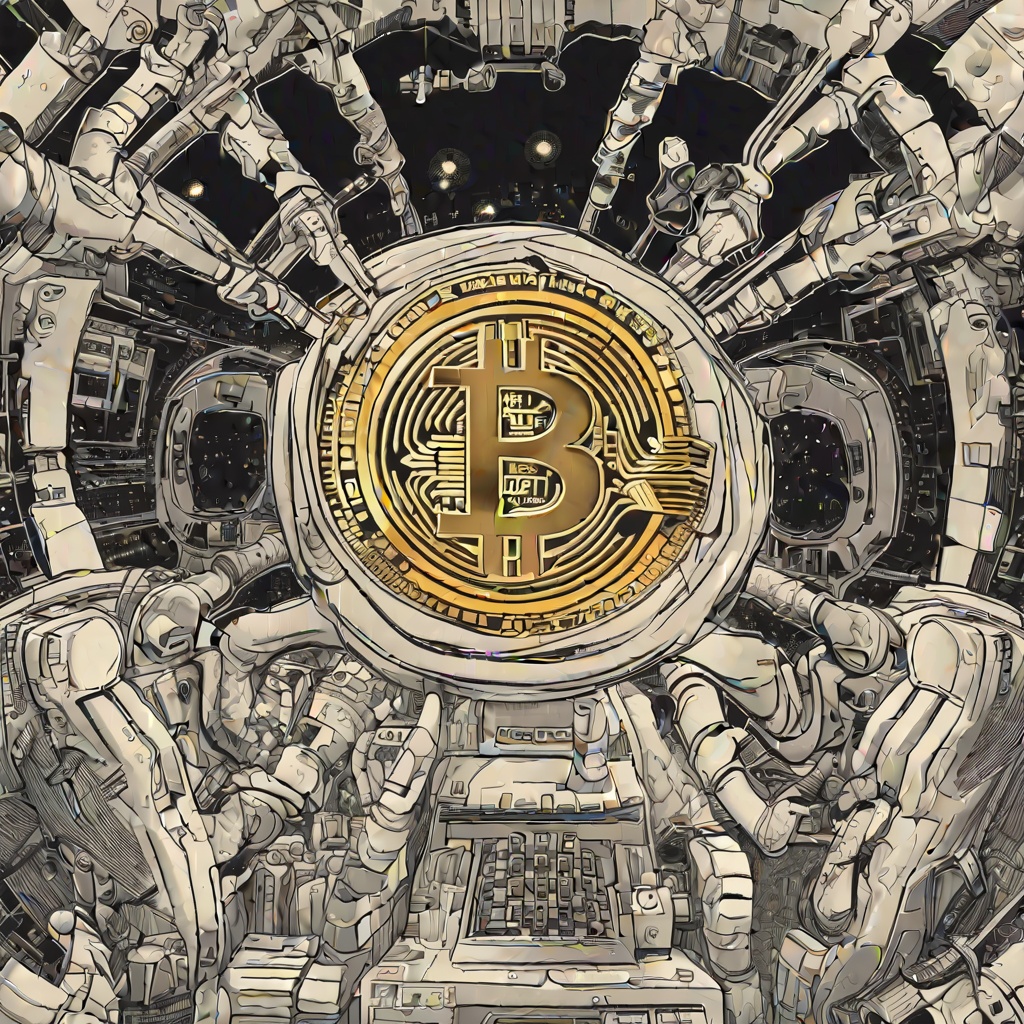