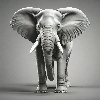
What are the disadvantages of SVM?
Can you elaborate on the drawbacks of using Support Vector Machines (SVM) in financial analysis and cryptocurrency predictions? Are there any limitations in terms of scalability, interpretability, or their ability to handle non-linear relationships effectively? Additionally, how might SVM's sensitivity to hyperparameters and the choice of kernel function affect the accuracy and stability of predictions in the volatile cryptocurrency market? Lastly, are there more suitable machine learning algorithms for this domain, and if so, why?
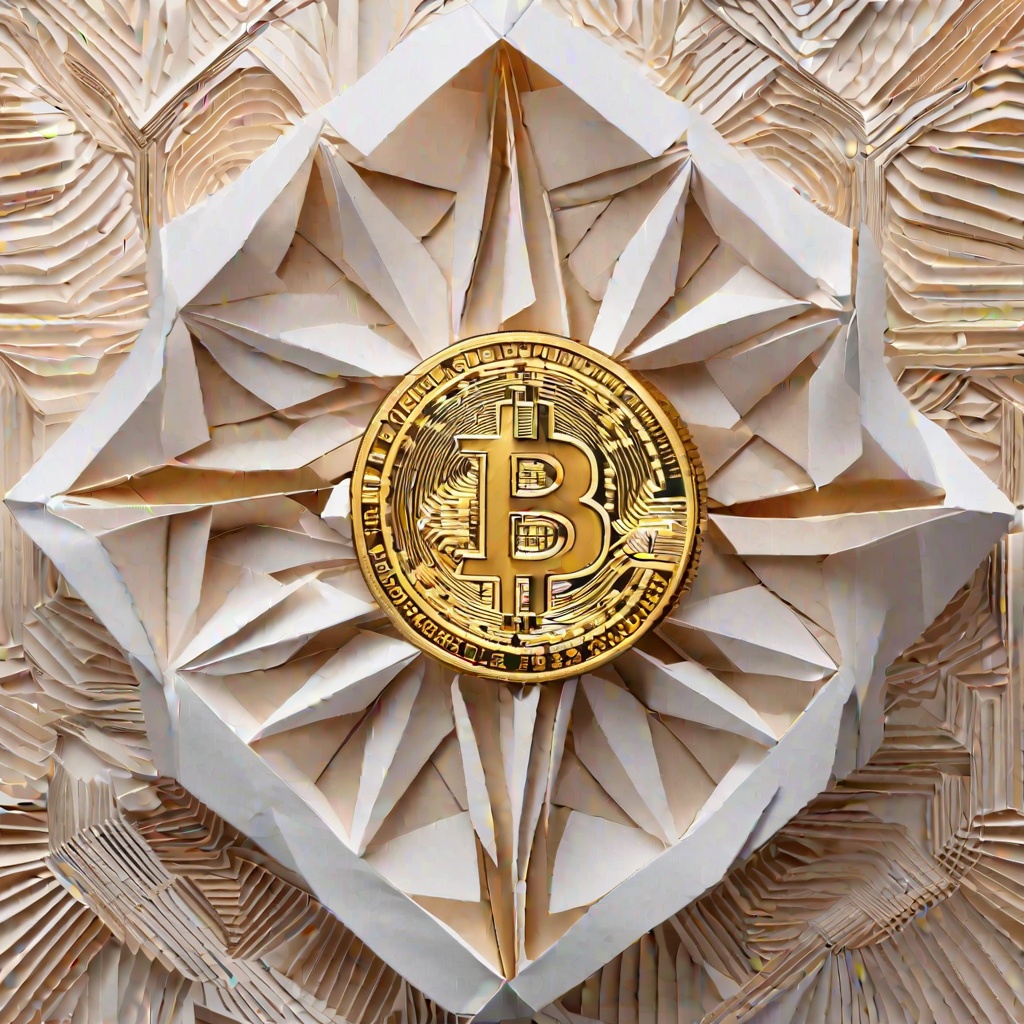
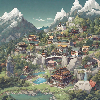
Why is SVM good for prediction?
Could you elaborate on why SVM, or Support Vector Machine, is considered a good choice for prediction tasks? It's my understanding that SVMs work by finding a hyperplane that separates data points into distinct classes, but how does this translate into accurate predictions? Are there specific properties of SVMs that make them more effective than other machine learning algorithms for prediction? Additionally, are there any limitations or scenarios where SVMs may not be the best choice for prediction?
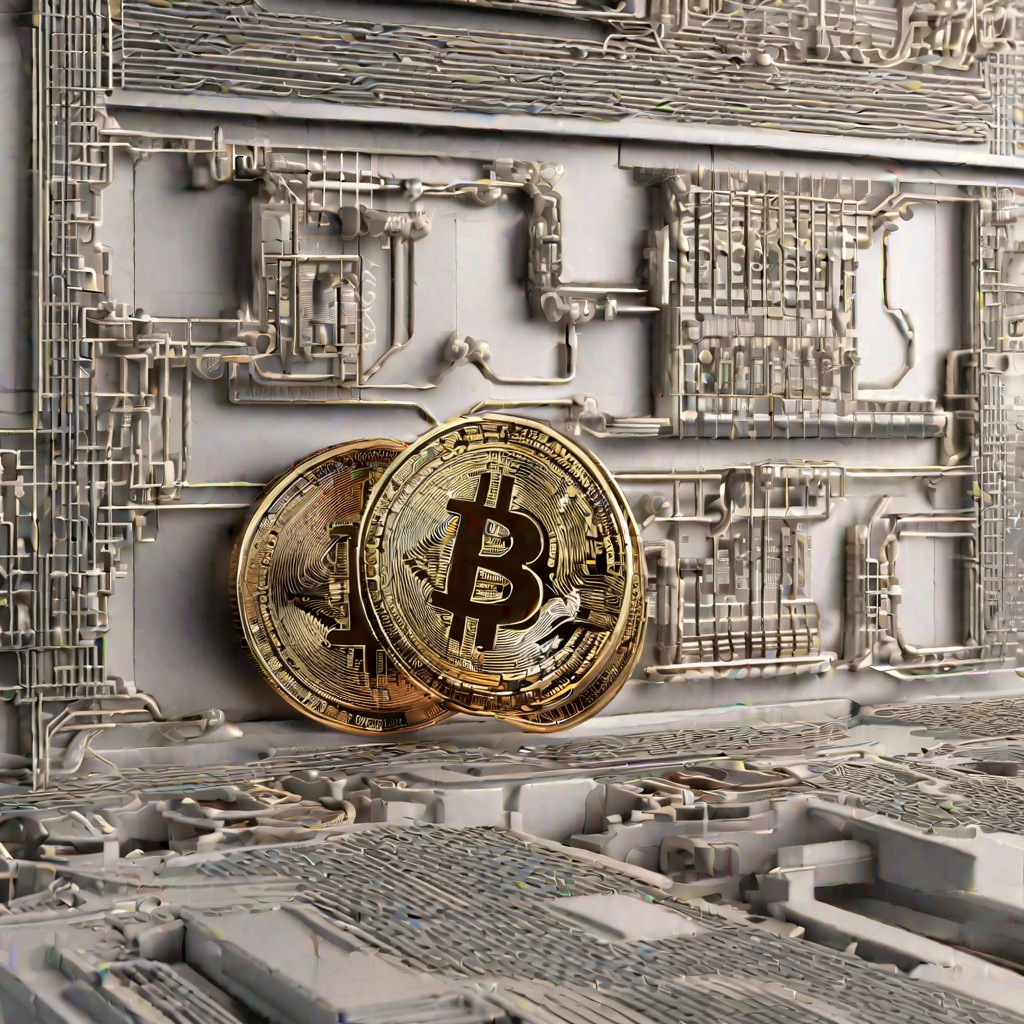
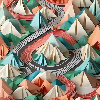
What is the price prediction for SVM?
Could you please elaborate on your thoughts regarding the future price prediction for SVM? Are there any specific factors or trends that you believe will influence its value in the coming months or years? Additionally, how do you compare SVM's potential growth to other cryptocurrencies in the market, and what strategies would you recommend for investors looking to capitalize on potential price movements?
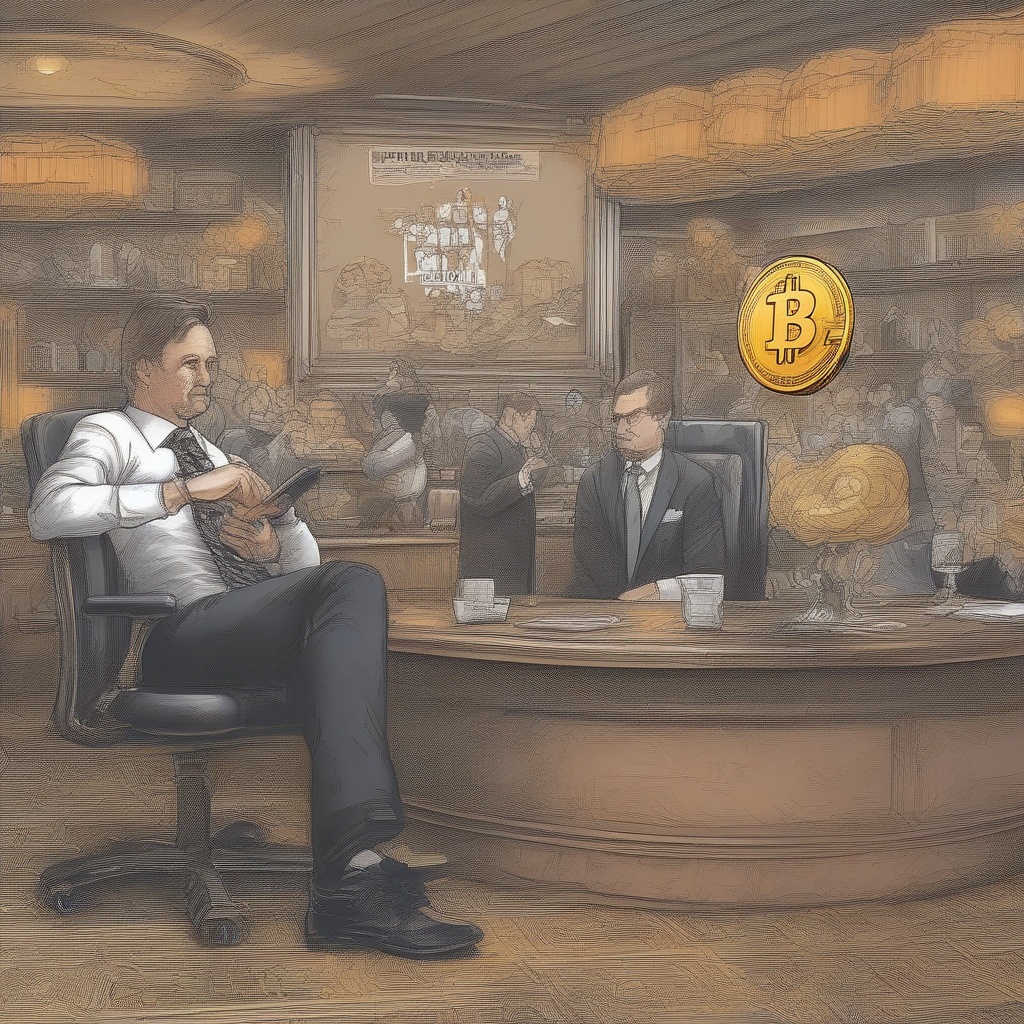
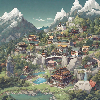
Who invented SVM?
Who exactly was the visionary behind the creation of Support Vector Machines (SVM)? Was it a solitary genius who toiled away in solitude, or was it a collaborative effort of a team of experts? Did the concept of SVM emerge from the depths of academic research, or was it sparked by a practical need in the industry? The history behind the development of SVM is fascinating, and I'm eager to know more about the person or persons who brought this powerful machine learning tool into existence.
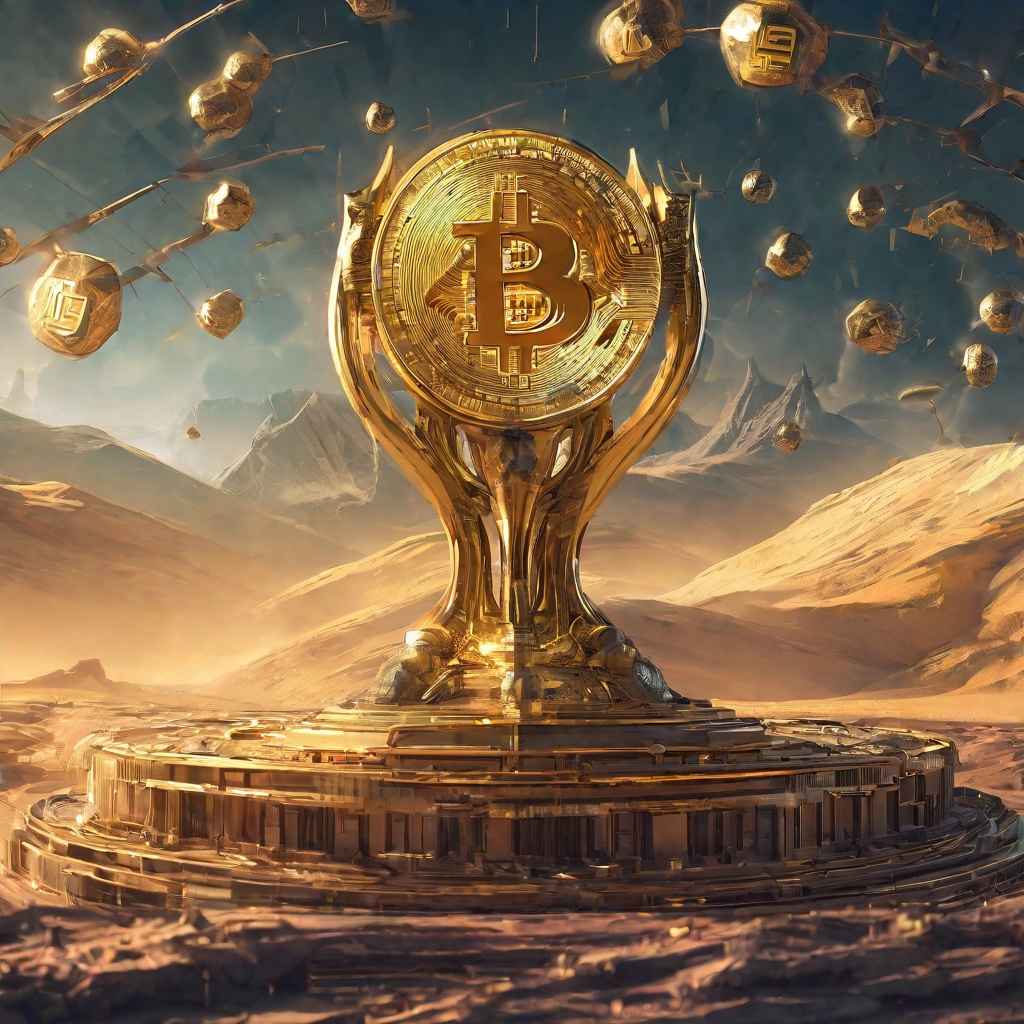
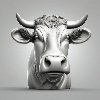
When should you use SVM?
Could you elaborate on the scenarios where Support Vector Machines (SVM) would be the most suitable choice for a machine learning task? Are there specific types of data or problems that SVM tends to excel at solving? Additionally, what are some of the potential drawbacks or limitations of using SVM that practitioners should be aware of before implementing it in their projects?
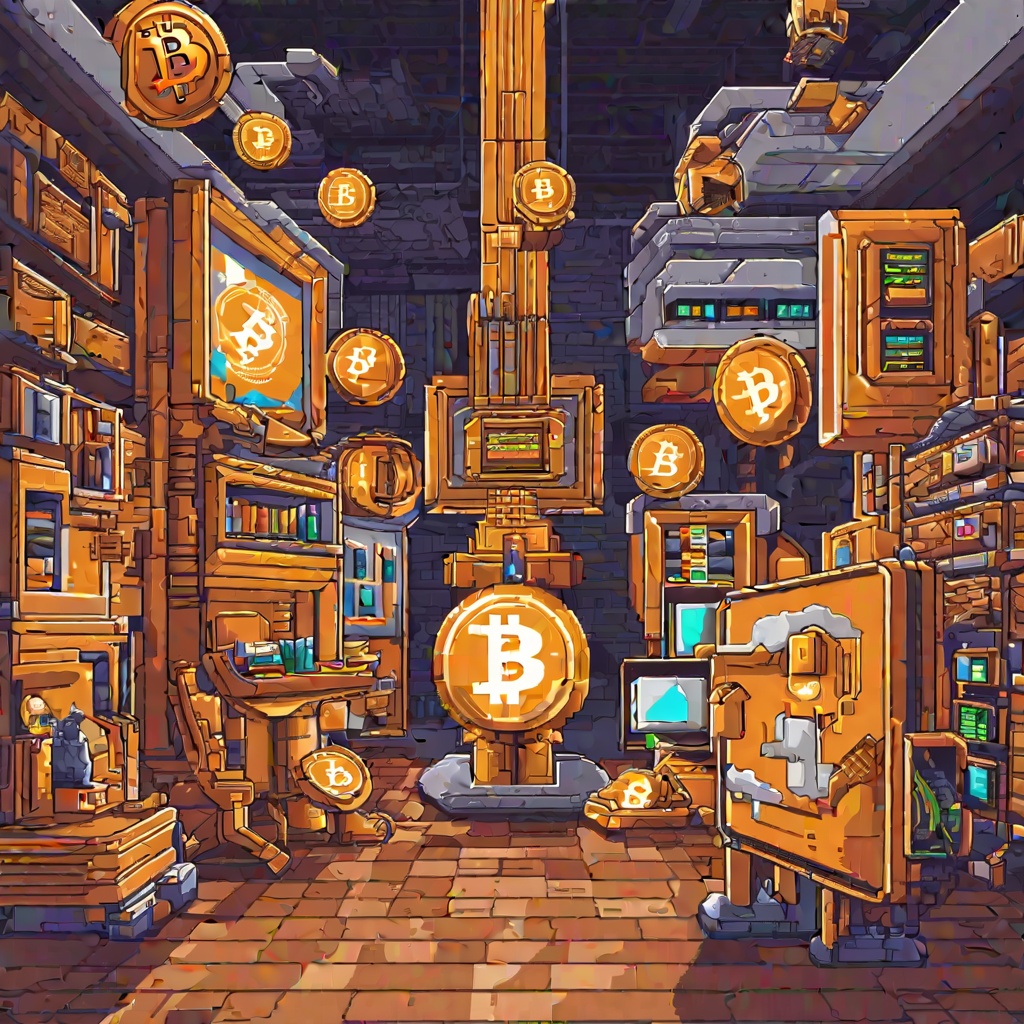