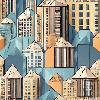
Why is GMM useful?
GMM is useful because it provides a flexible framework for modeling complex data distributions. By assuming that the data is generated from a mixture of multiple Gaussian distributions, GMM can capture more nuanced patterns and structures in the data, leading to improved performance in various machine learning tasks such as clustering and density estimation.
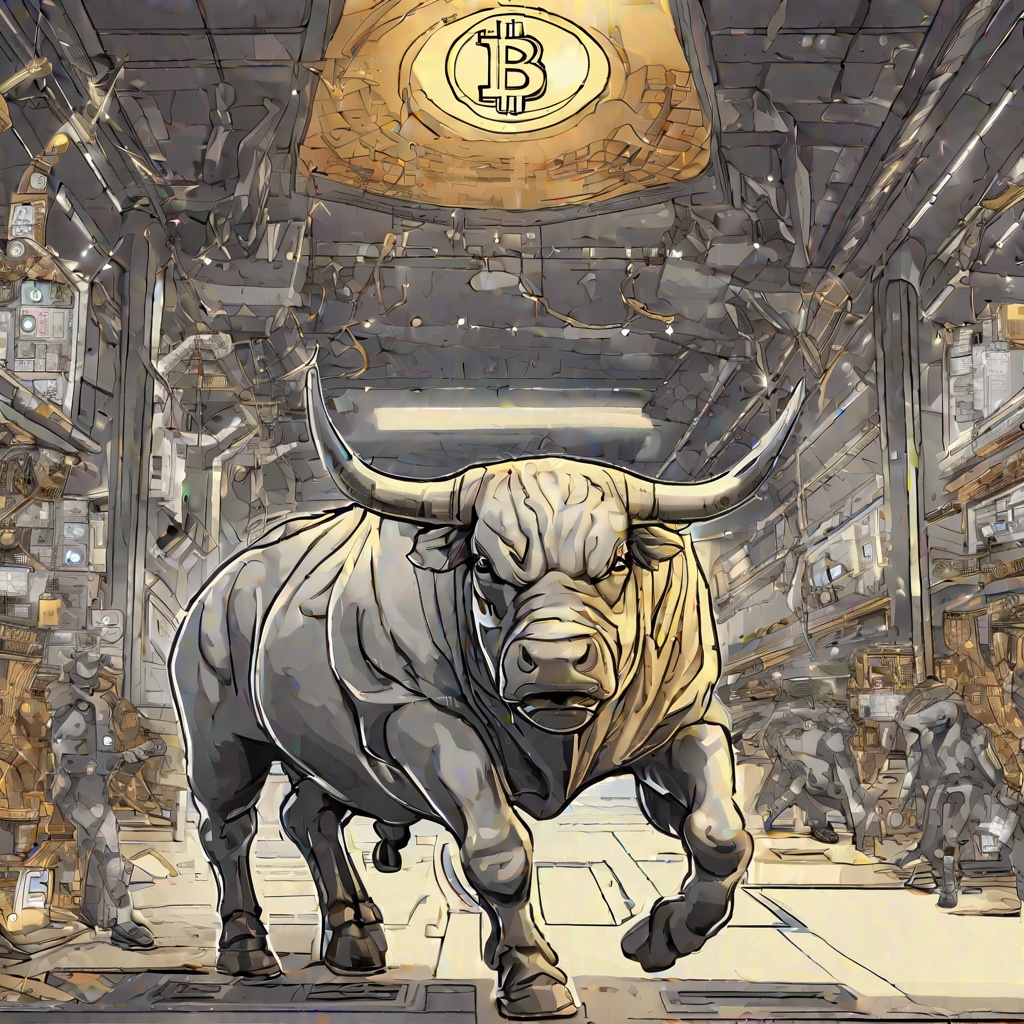
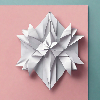
When should I use GMM?
I'm wondering about the appropriate situations to apply Gaussian Mixture Model. Specifically, under what circumstances or for what types of problems would GMM be the most suitable choice?
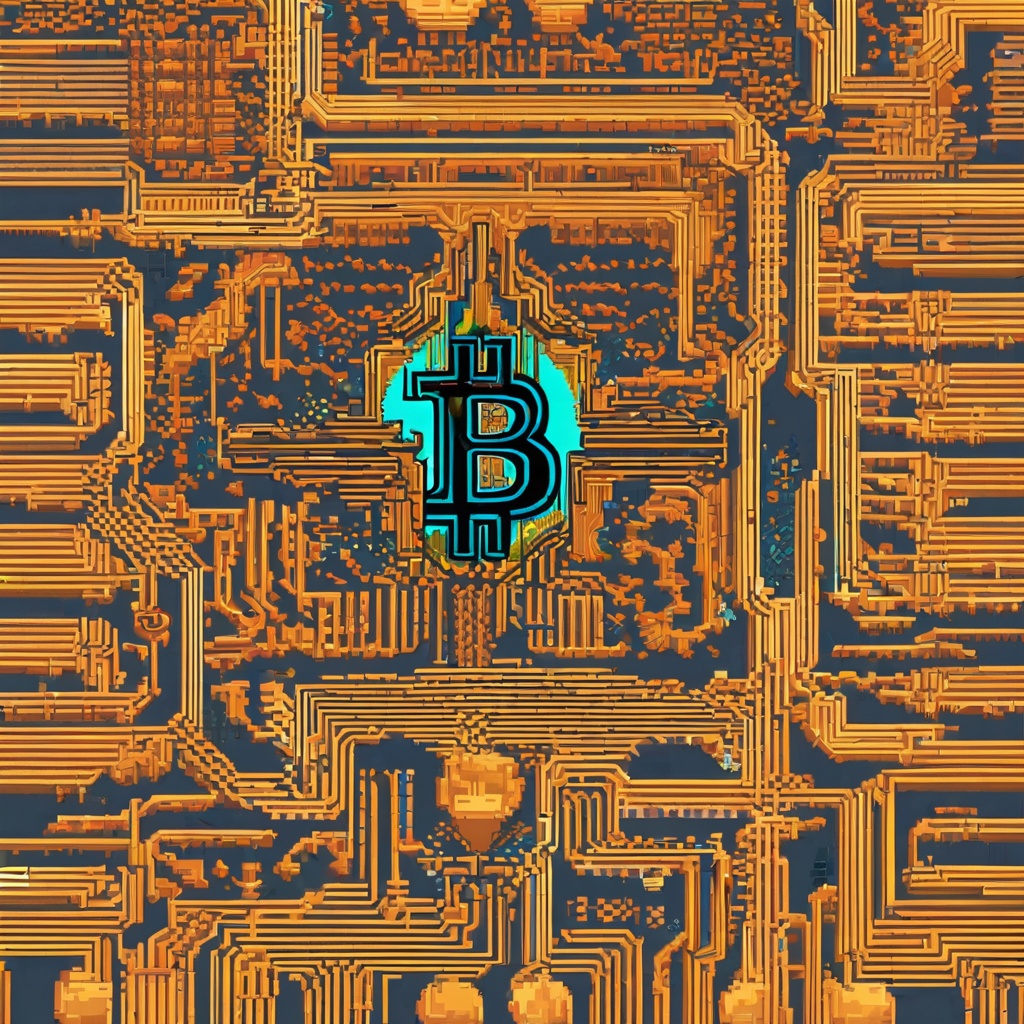
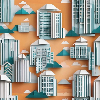
What's the full meaning of GMM?
I'm trying to understand the complete meaning of the acronym 'GMM'. Could you please explain what it stands for and its full significance?
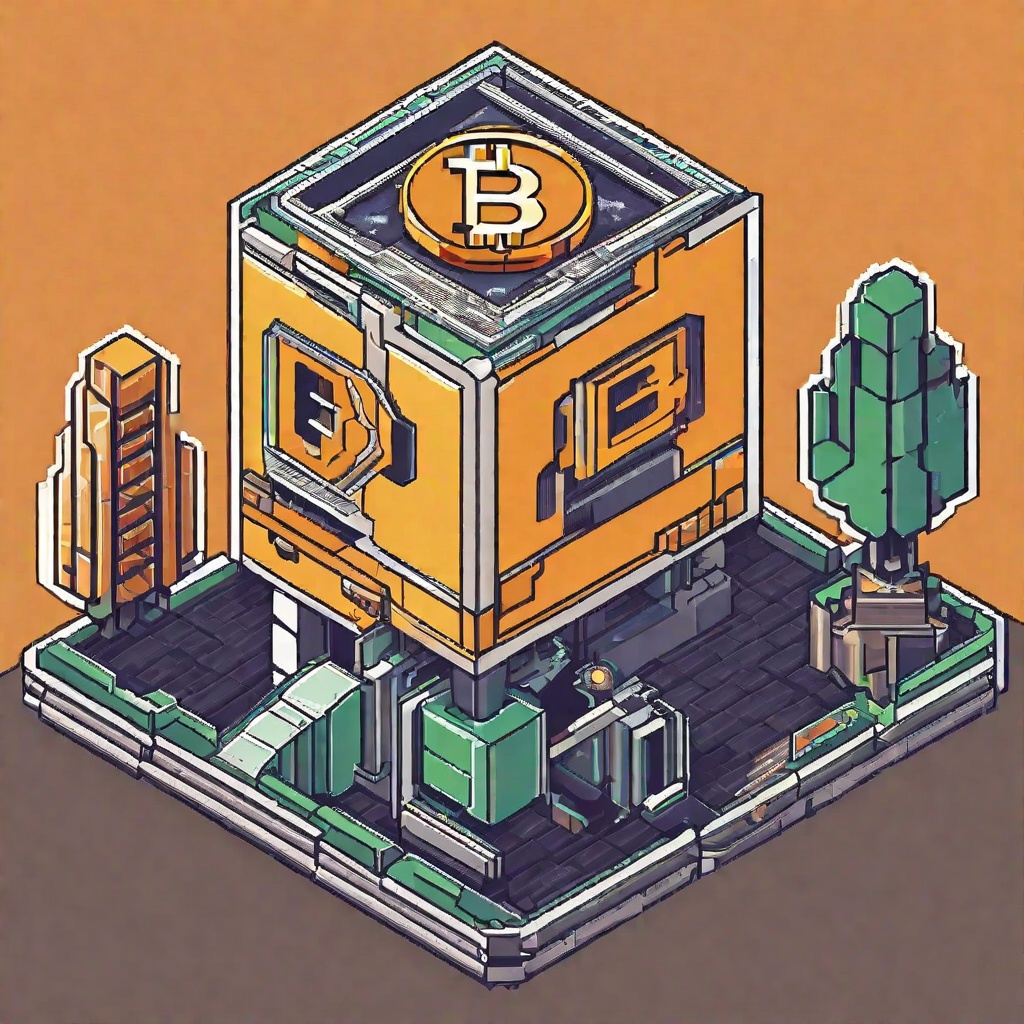
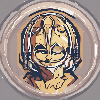
Why is GMM important?
GMM is crucial as it provides a flexible framework for statistical modeling, allowing complex data distributions to be accurately represented. Its importance lies in its ability to capture the underlying structure of data, essential for various applications like speech and image recognition.
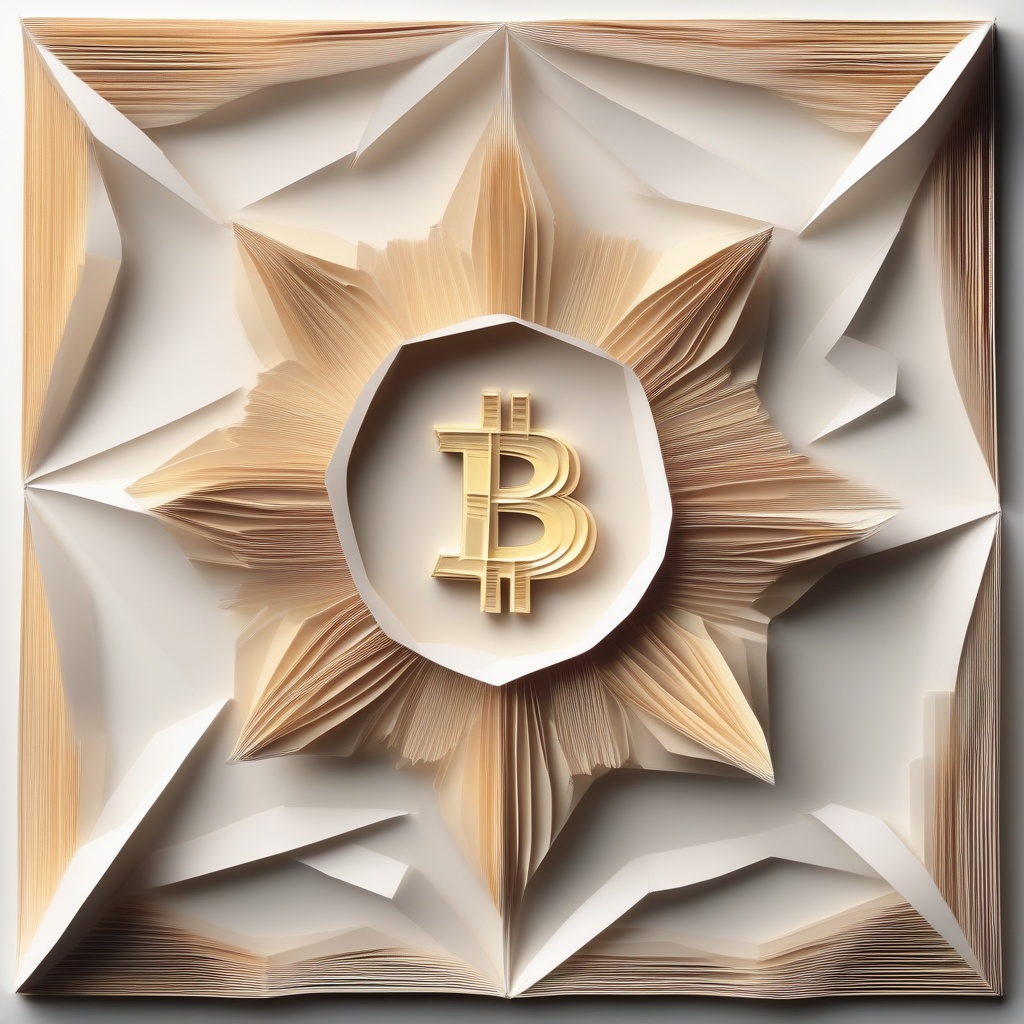
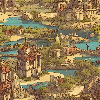
What is the limitation of GMM?
I'm interested in understanding the limitations of Gaussian Mixture Models (GMM). What are the constraints or restrictions that I should be aware of when using this model?
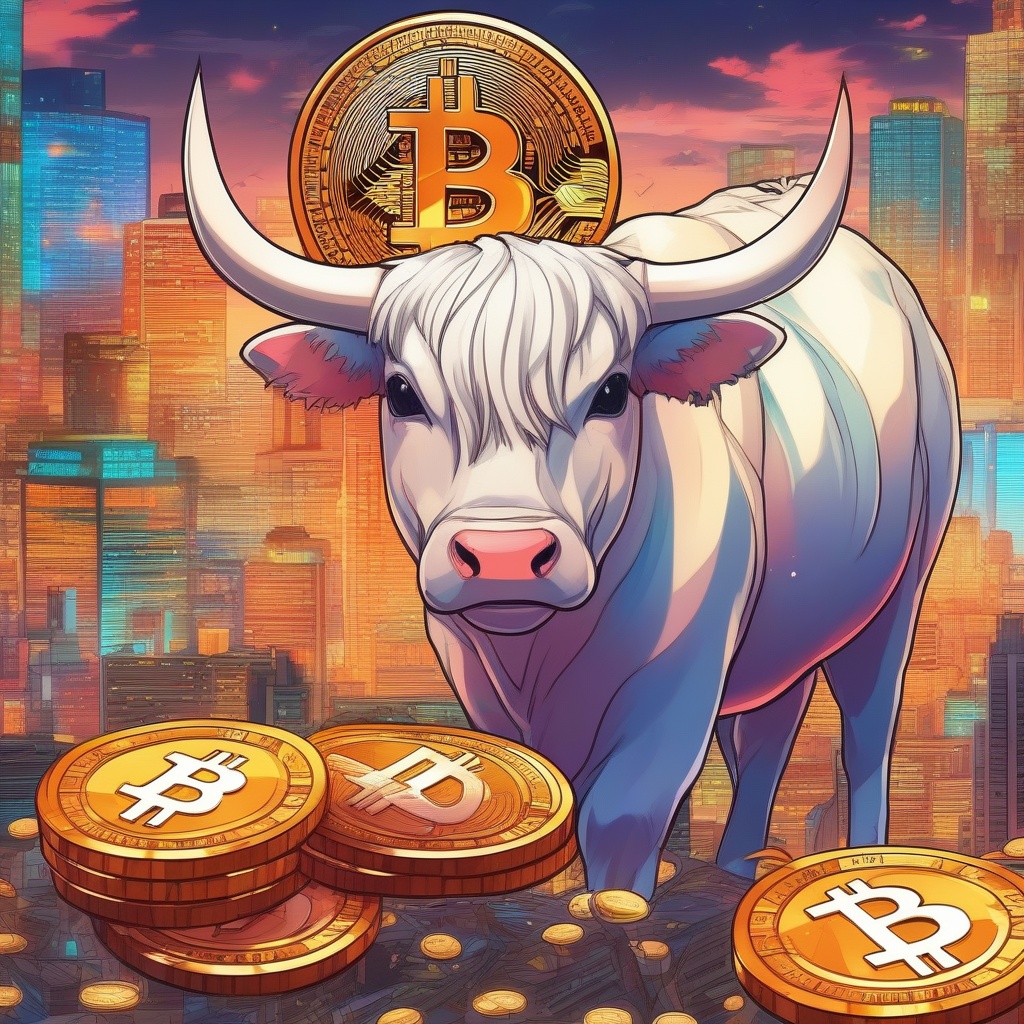